In today’s fast-paced electronics manufacturing landscape, optimizing test processes is paramount to achieving higher yields, reducing costs, and accelerating time-to-market. The advent of generative data paired with advanced analytics has ushered in a new era of possibilities for manufacturers. By harnessing the power of these technologies, businesses can gain unprecedented insights into their test and manufacturing processes, identify areas for improvement, and drive significant efficiency gains.
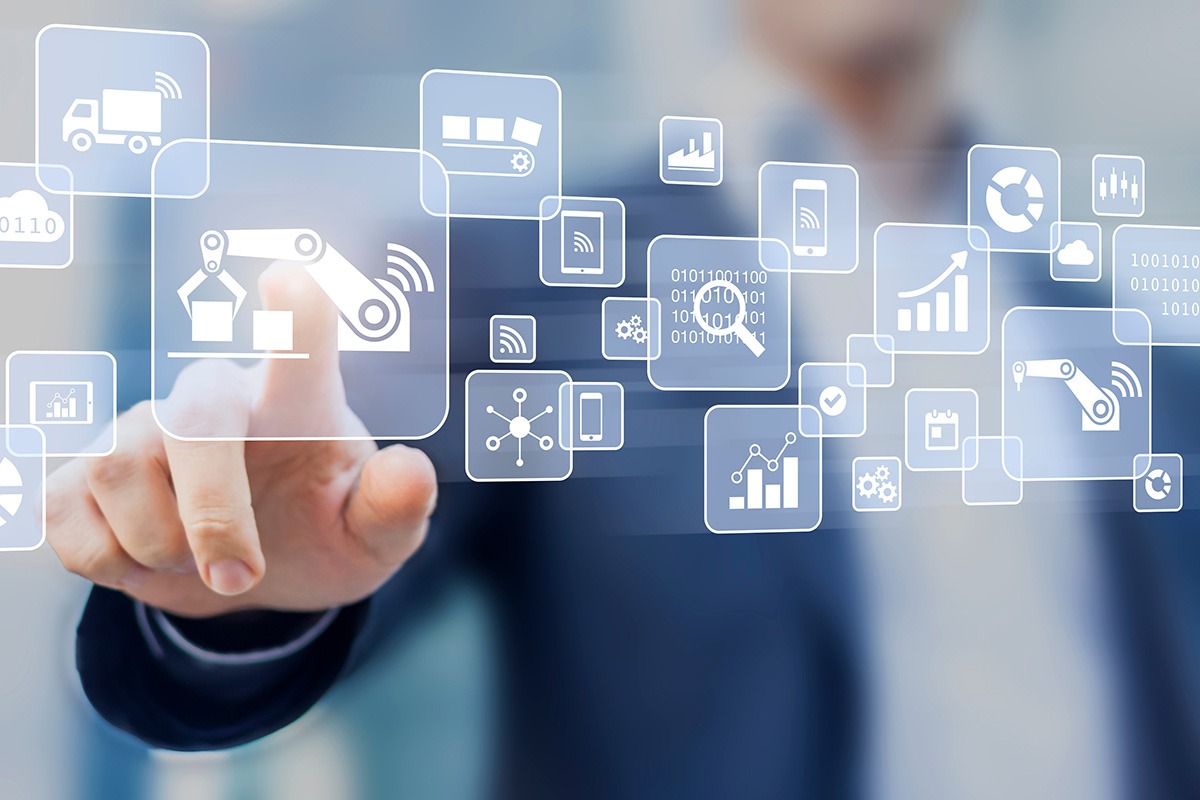
The Role of Generative Data Analytics
Generative data analytics, a subset of artificial intelligence, focuses on creating new data instances based on existing patterns. In the context of test processes, this means generating synthetic test data to augment real-world data sets. By doing so, manufacturers can:
- Enhance data quality: Fill in gaps in existing data and improve data analysis accuracy.
- Increase data volume: Generate larger datasets to train more robust machine learning models.
- Protect sensitive information: Fill in gaps in existing data and improve data analysis accuracy.
Leveraging Analytics for Process Optimization
Once generative data analysis is integrated into a test process, advanced analytics can be employed to extract valuable insights. Key analytical techniques include:
- Predictive analytics: Forecast potential issues and failures, enabling proactive maintenance and troubleshooting.
- Prescriptive analytics: Recommend the best test parameters and adjustments based on real-time data analysis.
- Root cause analysis: Identify the underlying causes of test failures, leading to targeted and effective corrective actions.
By generating synthetic test data to complement existing datasets, electronic services can:
- Improve test coverage.
- Reduce test cycle time.
- Increase overall equipment effectiveness (OEE).
Generative data analtyics, characterized by its large volume, velocity, and variety, offers a transformative opportunity to revolutionize test processes. By collecting and analyzing data from various sources, including test equipment, production systems, and external factors, manufacturers can gain a comprehensive understanding of their operations.
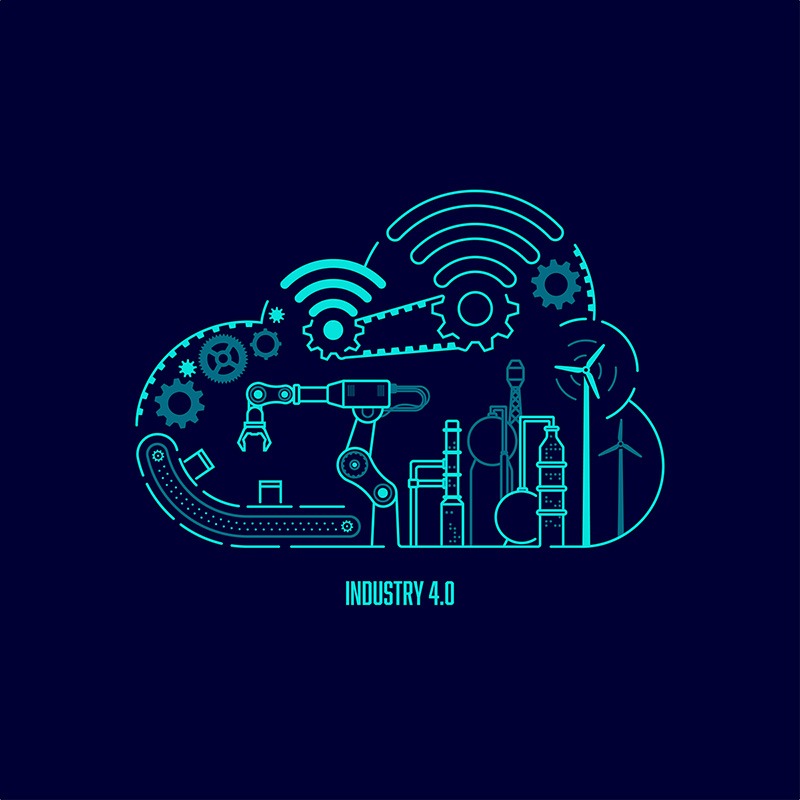
Data Collection:
Implementing advanced data capture systems enables real-time collection of test data, including test results, equipment and process performance metrics, and environmental conditions.
Data Cleaning and Preparation:
Using data cleaning and preprocessing techniques ensures data accuracy and consistency, preparing it for analysis.
Data Analysis:
Employing powerful data analytics tools, such as machine learning and artificial intelligence, allows for the identification of patterns, trends and anomalies within the test data.
Predictive Modeling:
Building predictive data analytics models based on historical patterns, enables manufacturers to anticipate potential issues and take proactive measures to prevent inefficiencies, downtime and unanticipated failures.
Prescriptive Analytics:
Utilizing prescriptive analytics provides actionable recommendations to optimize test parameters, improve equipment utilization, and improve efficiencies.
By leveraging test data on manufacturing processes, advanced analytics can identify patterns and trends to predict equipment failures and prescribe test and manufacturing improvements with high accuracy. Proactive maintenance based on these predictions results in reduced downtime and increased overall equipment effectiveness (OEE).
The combination of generative data and analytics offers immense potential for transforming test processes and electronics design and manufacturing. By embracing these technologies, manufacturers can achieve unprecedented levels of efficiency, quality, and profitability.